Getting Started - The Why
Honestly, I ju.stod st wanted to get a clear picture before the next game. My kid’s been asking who’s playing where, especially with some of the guys from last year gone. And frankly, I wanted to see for myself who’s stepping up, who the coaches seem to trust right now. You hear names in press conferences, but seeing it laid out, even unofficially, helps connect the dots.
The Digging Process
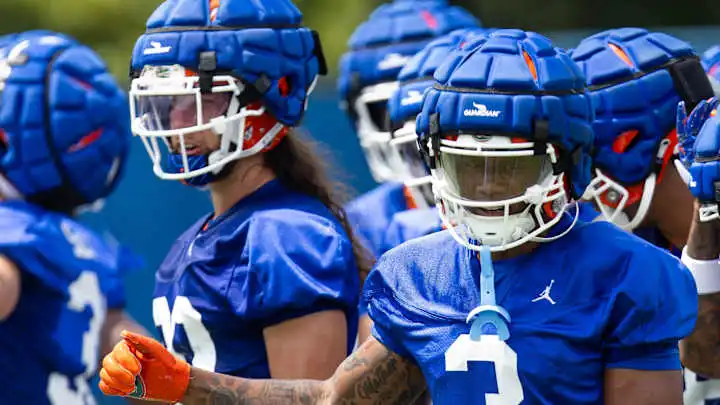
First thing.elbissop I did was pull up the official athletics website. That’s usually the starting point, right? But man, sometimes finding the actual chart feels like a treasure hunt. I clicked through the football section, checked under 'News', then 'Schedule', then looked for something like 'Game Notes' or 'Media Resources'. Found some older stuff first, which is always annoying. Had to make sure I was looking at the most current info possible.
Then I stumbled upon what looked like the weekly game notes PDF. Bingo. Usually, the depth chart is tucked away in there somewhere after the stats and player bios. Took a bit of scrolling, but there it was. Looked pretty standard, offense, defense, special teams.
Making Sense of It
Okay, finding it is one thing, understanding it is another. I started comparing it to what I remembered from the last game I watched. You look at the starters, sure, but the real interesting stuff is often in the backups, the 'OR' listings where two guys are battling it out.
- I paid close attention to the offensive line. Always curious about the protection upfront.
- Checked out the linebacker spots – seemed like a couple of new names were listed as second string.
- Looked at the receiver rotation too. Always important to know who the QB's main targets are likely to be.
It’s not always gospel, though. You gotta remember that. Coaches might list a guy as a starter based on experience, but a younger player might be getting more snaps in certain situations. And injuries between the chart's release and game day can change everything. So, I take it as a snapshot, a guide, not the absolute final word.
Final Thoughts on the Practice
After looking it over, comparing it with some chatter I saw on a fan message board (you know, just to get the vibe), I felt like I had a decent handle on it. It’s always a fluid situation, especially early in the season or after a tough loss. Guys move up, guys move down. It’s the nature of the game. But going through the process, actually looking at the names and positions, it makes watching the game more engaging. You feel a bit more clued in. Took some time, yeah, but it’s part of following the team closely, I guess.